AI and advanced nuclear reactors
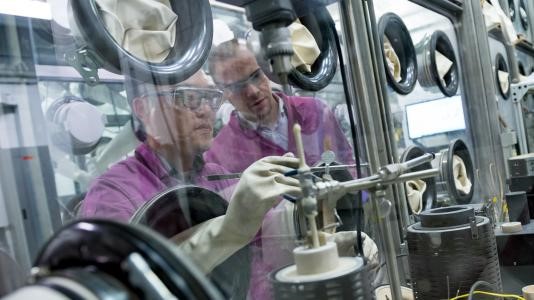
Scientists are searching for new materials to advance the next generation of nuclear power plants. In a recent study, researchers at the Department of Energy’s Argonne National Laboratory showed how artificial intelligence could help pinpoint the right types of molten salts, a key component for advanced nuclear reactors.
The ability to absorb and store heat makes molten salt important to clean energy and national climate goals. Molten salt can serve as both coolant and fuel in nuclear power reactors that generate electricity without emitting greenhouse gases. It can also store large amounts of energy, which is increasingly needed on an electric grid with fluctuating sources such as wind and solar power.
Salt pick: If you heated salt on your kitchen table up to 801 °C (1,474 °F), it would melt, and you’d have molten salt. However, for making and storing energy, not just any salt will do. Scientists are exploring different combinations of salts to get the exact properties needed to cool and fuel a nuclear power reactor efficiently for decades. These properties include, among others, lower melting temperatures, the right consistency, and the ability to absorb high amounts of heat.
Which molten salt blueprints will deliver the desired traits for a nuclear reactor? The potential variations are nearly endless. The study set out to determine whether computer simulations driven by machine learning could guide and refine real-world experiments at the Advanced Photon Source (APS), a DOE Office of Science user facility at Argonne. The results were recently published in the journal Physical Review B.
“We used experimental results from the APS to validate our simulation. At the same time, the simulation results provided us more details about which salts to study further. They work with each other,” said Jicheng Guo, a chemical engineer at Argonne and the paper’s lead author. “This allows us to study multiple compositions at the same time.”
APS work: Researchers use the powerful X-rays at the APS to better understand specific salt mixtures by looking closely at their structures. But the time and cost associated with real-world experiments make it desirable to narrow the field of candidates that undergo inspection.
“The possible composition space for molten salts is enormous,” said Nathaniel Hoyt, an Argonne researcher and coauthor of the paper. “So, it would be impossible to try to take experimental data for every possible composition.”
At the facility’s 6-ID-D beamline, a technique called high energy X-ray diffraction captures the patterns generated as the X-ray beams scatter off a sample of molten salt.
“APS is unique for these types of measurements,” said Chris Benmore, a senior physicist at the APS and coauthor of the paper. “The high energy X-rays it generates are very good for looking at the structure of molten liquids, glasses and amorphous materials in general.”
Active learning: Machine learning involves training a computer to analyze a situation based on existing data. But in this case, the researchers did not have an abundance of validated examples to show the model. Building on previous modeling that explored heat-resistant materials, researchers used what’s known as active learning to create a transferable model to analyze molten salts.
Rather than being fitted for one or two specific molten salt mixture compositions, the transferable model can be applied to mixtures across the composition space. The model makes predictions based on principles rather than a set of predefined answers. The machine learning simulations were run using high performance computing resources at the Argonne Leadership Computing Facility (ALCF), a DOE Office of Science user facility, using the Bebop cluster at Argonne’s Laboratory Computing Resource Center.
“We didn’t train the model with examples of that sweet-spot composition, where you get the right melting point,” said Ganesh Sivaraman, an Argonne computational scientist and the paper’s corresponding author. “Our model managed to predict that sweet spot, even without the corresponding training input.”
Now that the researchers have shown this approach can work, the next step is to work with even more complex data.
“A molten salt reactor is quite a dynamic environment. The conditions change over time, and sometimes impurities can get into the salt,” Guo said. “We want to introduce a tiny amount of these impurities to see whether the model can predict how that affects the overall structure of molten salts and their properties.”
Office of Science and DOE’s Advanced Scientific Computing Research program supported the research. Coauthors with Guo, Hoyt, Sivaraman, and Benmore are Logan Ward, Yadu Babuji, Mark Williamson, and Ian Foster of Argonne and Nicholas Jackson of the University of Illinois–Urbana-Champaign.