AI accelerates search for safer, more durable materials for nuclear reactors
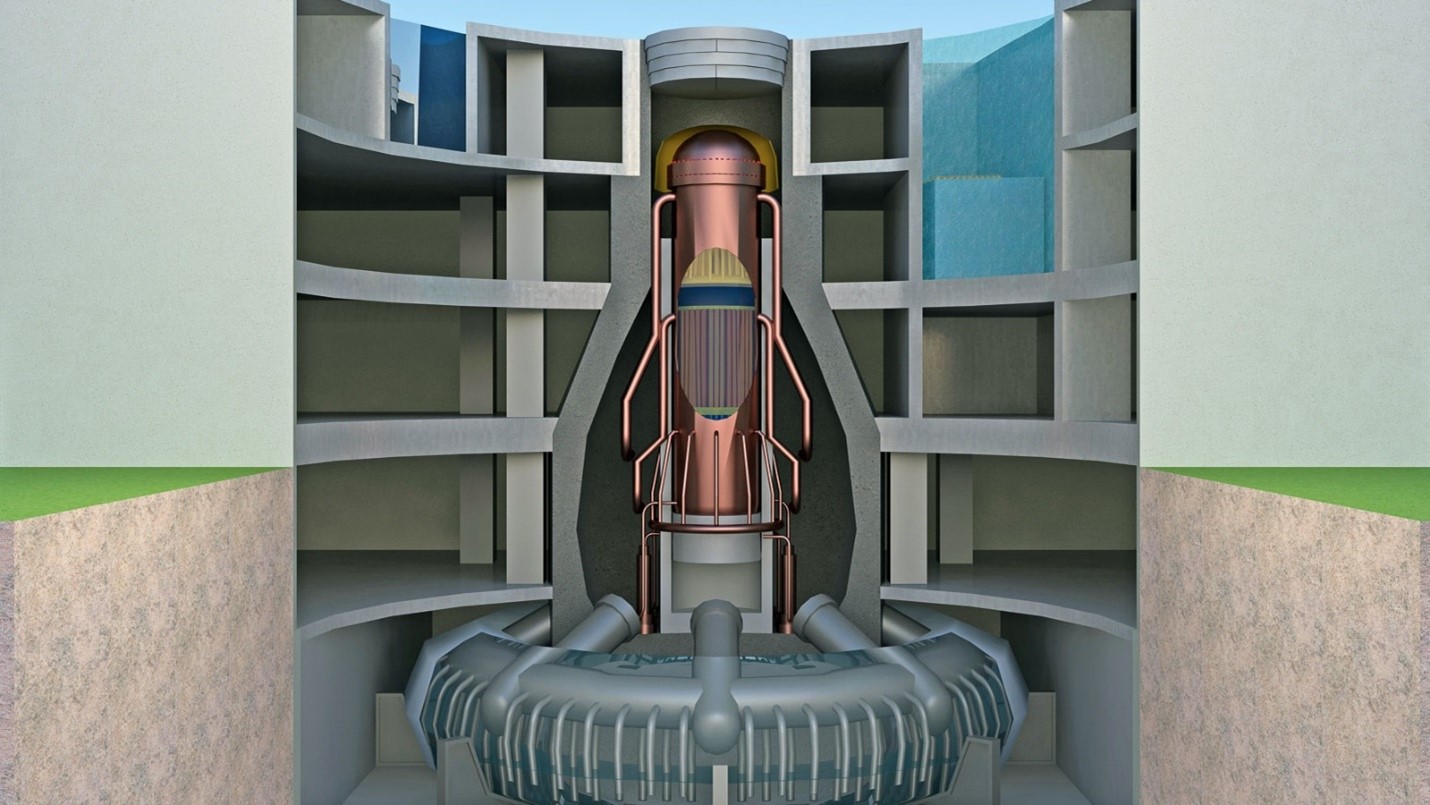
Researchers from the Department of Energy’s Argonne National Laboratory are developing a “tool kit” based on artificial intelligence that will help better determine the properties of materials used in building a nuclear reactor.